Can you please introduce yourself?
I am the founder of the Predictive Analytics World conference series, executive editor of The Predictive Analytics Times, and author of “Predictive Analytics: The Power to Predict Who Will Click, Buy, Lie, or Die,” a former Columbia University professor and a speaker educator, and consultant.
More information about myself, the book, and the conference series can be found at www.thepredictionbook.com.
What is predictive analytics? How do you define it, and how did you get interested in working with predictive analytics?
As defined in my book, “Predictive Analytics,” this is technology that learns from experience (data) to predict the future behavior of individuals in order to drive better decisions.”
I’m a former academic and in that life I was interested in the core science, machine learning. Getting a computer to learn from examples (experience) is the most interesting thing in science, to me. It is also incredibly powerful.
As a result, predictive analytics affects everyone every day. Trendsetters like Chase, Facebook, Google, Hillary for America, HP, IBM, Match.com, Netflix, the NSA, Pfizer, Target, and Uber are seizing upon the power of big data to predict human behavior—including yours. Why? Predictive analytics reinvents industries and runs the world. It combats risk, boosts sales, fortifies healthcare, optimizes social networks, toughens crime fighting, and wins elections.
What have been some of the main advances that have fueled the rise of predictive Analytics in recent years?
Ensemble models (Chapter 6 of my book) and uplift modeling (Chapter 7). They are game changers.
Ensemble models are the most prevalent way to advance from simple methods like decision trees in order to improve predictive performance. As with most advanced method, you lose transparency (model interpretability) — but you don’t lose all that much simplicity: an ensemble model is nothing more than a collection of models such as decision trees that vote (or are otherwise combined). This is kind of like collective intelligence — the wisdom of a crowd not of people but of predictive models.
Uplift modeling is the move from predicting what will happen (standard predictive analytics) to predicting whether a treatment choice (marketing or healthcare, for example) will result in the positive, desired outcome. US President Obama’s 2012 campaign used this method to optimize voter contact. A good starting point on this topic is my article, “Personalization Is Back: How to Drive Influence by Crunching Numbers“.
How is predictive analytics and machine learning related?
The two are largely synonymous. In academics and R&D labs, it is called “machine learning” (although that is a slightly broader area), and when deployed commercially, it is “predictive analytics.”
This makes sense, since the machine needs to learn in order to predict. It learns from data how to consider the factors (variables) known about an individual in order to predictively score that individual. The mechanism that considers multiple factors to derive a single score, e.g., a decision tree or neural network, is called a predictive model; the process of creating the model from data – modeling – is the learning process, and the model is what’s been learned.
What industries are investing heavily in predictive analytics?
Most are. For example, we have launched industry-focused Predictive Analytics World events for several: PAW Business, PAW Government, PAW Healthcare, PAW Manufacturing, and PAW Workforce. See more info at pawcon.com.
What challenges do you see for young entrepreneurs to start their predictive analytics company?
If someone is starting a new company in this space, there are many opportunities. However, there are many players so a lot of homework must be done to define your point of differentiation.
OTOH, if someone is starting anew applying predictive analytics within their job/organization, getting started is straight forward. We have a Predictive Analytics Guide for that purpose: pawcon.com/guide.
What personal/professional projects have you been working on this year, and why/how are they interesting to you?
I’m currently finishing the Revised and Updated edition of the book “Predictive Analytics.” It includes dozens of new examples from Facebook, Hopper, Shell, Uber, UPS, the U.S. government, and more. Its compendium of mini-case studies has grown to 182 entries, including breaking examples.
The new edition also introduces a section on the “real” reason the NSA wants US citizen data: Automatic Suspect Discovery. In the writing, I presume—with much evidence—that the National Security Agency considers predictive analytics a strategic priority. I address the questions, can the organization use PA without endangering civil liberties?
Updating this accessible book is exciting to me because the predictive analytics industry continues to take off. Accenture and Forrester both report that PA’s adoption has more than doubled in recent years. Transparency Market Research projects the PA market will reach $6.5 billion within a few years. A Gartner survey ranked business intelligence and analytics as the current number one investment priority of CIOs. And in a Salesforce.com study, PA showed the highest growth rate of all sales tech trends, more than doubling its adoption in the next 18 months. High performance sales teams are four times more likely to already be using PA than under performers.
What inspired you to write “the power to predict who will click, buy, lie or die“. Who should read your book?
The book is for everyone. It’s easily understood by all readers. Rather than a how-to for hands-on techies, the book serves lay readers, technology enthusiasts, executives, and analytics experts alike by covering new case studies and the latest state-of-the-art techniques.
I wrote this book to demonstrate why PA is intuitive, powerful, and awe-inspiring. It’s a book about the most influential and valuable achievements of computerized prediction, and the two things that make it possible: the people behind it, and the fascinating science that powers it.
While there are a number of books that approach the how-to side of predictive analytics, this book serves a different purpose (which turned out to be a rewarding challenge for its author): sharing with a wider audience a complete picture of the field, from the way in which it empowers organizations, down to the inner workings of predictive modeling.
With its impact on the world growing so quickly, it’s high time the predictive power of data—and how to scientifically tap it—be demystified. Learning from data to predict human behavior is no longer arcane.
What tips would you like to share with someone starting out in predictive analytics?
There are many great resources for learning and getting started, including videos, conferences (including the series I founded, Predictive Analytics World), books, articles, and training programs (including my online course at www.businessprediction.com). We’ve put together the Predictive Analytics Guide to summarize these resources – www.pawcon.com/guide.
Start first with our Predictive Analytics Guide: www.pawcon.com/guide.
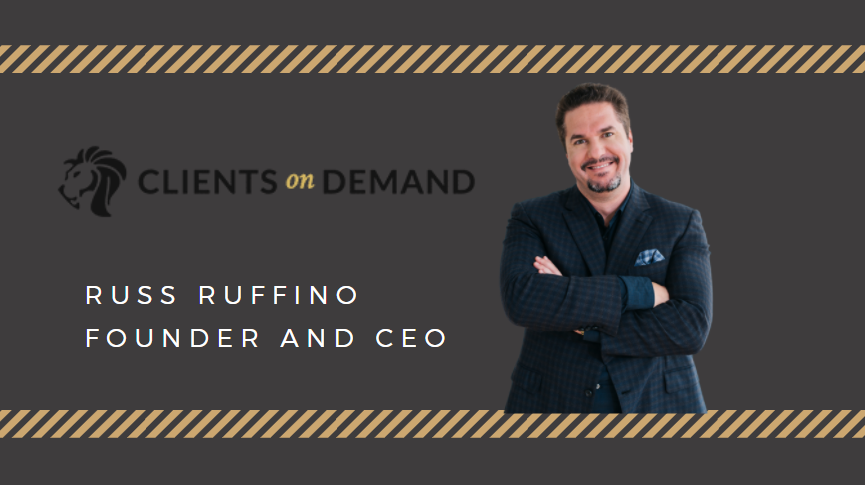
Interview with Russ Ruffino, CEO of Clients on Demand, on Customer Acquisition and Adopting a Growth Mindset
In this...