This is the ninth instalment in the series of essays on Statistics Denial by Randy Bartlett, Ph.D. To read other articles in the series, click here.
Myth #5: For a large number of observations (Big Data: Volume), all the variables are significant so statistics does not work.
Myth #6: Statistics does not accommodate ‘consequential’ statistical significance.
Myth #5 builds upon the old confusion around significance testing that comprises this second ‘ancient’ myth (#6). Suppose that you are building a predictive model based upon a billion observations (n) and using 500 variables (p). After you press the magic ‘make model’ button, all of the parameters are ‘significantly different’ … from zero. Conclusion, statistics does not work … WRONG.
Here is a quote typifying the misunderstanding:
‘“One big reason [why statistics does not work]… is that everything passes statistical tests with significance,” he says. “If you have a million records, everything looks like it’s good [significant].”’ According to the same person, ‘there’s a difference between statistical significance and what he calls operational [consequential] significance.’
First, if you are building predictive models, then why are you using hypothesis testing? Second, why are you using hypothesis testing? Third, if you must use hypothesis testing, then try the right one. Use the ‘new’ breakthrough from Neyman-Pearson (ca 1932), which addresses (consequential) statistical significance. Now we will be more specific about these three disconnects.
First, if you are building predictive models, then why are you using hypothesis testing?
For predictive models, whether coefficients are significantly different from zero is not the primary consideration. The point is whether the model predicts. We know, you seek parsimony by dropping parameters, which have coefficients that are not significant from zero. Hint: There are better statistical avenues to parsimony; use statistics designed for that task.
Recall that there are four modeling objectives: coefficient estimation, prediction, grouping, and ranking. Hypothesis testing was conceived for decision making largely in the context of coefficient estimation. As such, it is only an important sideshow to the main show of statistics—the logic of numbers with uncertainty.
Second, why are you using hypothesis testing?
Confidence intervals generally have more utility than hypothesis tests. We know, sometimes you just want or need a hypothesis test, yet not for prediction. Also, confidence regions nicely address multidimensional needs.
Third, if you must use hypothesis testing, then try the right one. Use the new breakthrough from Neyman-Pearson (ca 1932), which addresses (consequential) statistical significance.
Now we have arrived at Statistics Denial Myth #6, the old confusion between the Fisherian school of hypothesis testing and Neyman-Pearson. Fisher was the first to address the matter of hypothesis testing and he developed a logical approach, which compares unknown parameters to zero. Neyman-Pearson hypothesis testing famously expands this work by insisting on an alternative hypothesis and adding a term, δ , as a cutoff for (consequential) statistical significance. This has been called practical significance, economic significance, etc. and now operational significance. This portmanteau hypothesis test allows coefficients to be compared to any value, δ .
For example, suppose that if a coefficient exceeds some consequential value δ , then retaining it is statistically significant. The hypotheses might take the following form:
where δ is the cutoff for a consequential difference. Neyman would say that the alternative hypothesis, δ , should represent the consequential scenario. (See ‘Encyclopedia of Research Design,’ Vol. I, SAGE (2010), p. 298). Hence, consequential significance is statistical significance. As always, see a professional for your advanced statistics needs.
Close:
Confusion about hypothesis testing is completely understandable, yet not acceptable for self-professed experts.
While these misunderstandings have an amusing side, they also have an edge. At the extreme, we have seen hucksters broadcasting mischaracterizations of statistics to better position their lesser qualifications or to blunt legitimate criticism of their blatant mistakes. One common claim coming from hucksters innocent of statistics is that we do not need statistics anymore because we have access to them.
In Blogs 2 & 3, we discussed the harm caused by promotional hype extreme enough to adulterate statistics and circumvent best practice—our best tools for extracting the information.
We sure could use Deming, right now. Many of us who embrace the explicit rigorous logic and protocols of these tenets of data analysis hang out in the new LinkedIn group, About Data Analysis. Come see us.
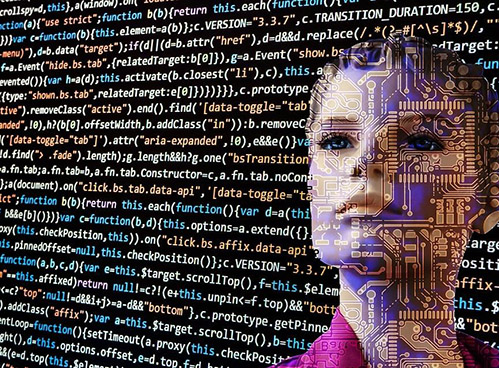
The data analytics of substance abuse & treatment
The...